PCA is just not very useful for image compression.
You're asking the question:
Why is it not used?
without first considering the question
Why should it be used?
You owe it to yourself (and honestly, to people trying to answer the question "why not?") to think about how it could be a good tool for compressing still images.
That's not clear, and I'll explain why I think you won't find a reason why it should be used:
What you want in (lossy) data compression is:
- identify features of the data that are more important for the purpose (here: visual perception by humans) than others
- decompose the image into coefficients to these features, assigning a lower data rate to the unimportant features
- arrange the resulting data in a way that is most useful for an entropy coder (e.g. LZW, LZMA)
The problem with PCA in this context is that the components you find are unknown to the receiver of an image – you need to send them as well.
So, to send an image $D$ (or a block in an image) with $N$ pixels, you first use PCA to find principal components $C_i$ (say, $m$ relevant principal components, $i=0,\ldots,m-1$), which each having $N$ pixels.
$$ D = \sum_{i=0}^{m-1} \alpha_i C_i$$
Now, you made $N\cdot m$ pixels out of your $N$ pixels file, plus $m$ coefficients $\alpha_i$. So, you greatly increased the amount of data you need to transport, instead of reducing it!
Even in the most extreme case, an image literally just containing a single principal component and nothing else, you would have not have gained anything – the component would be the original pixels, and not any better or worse to compress.
Other transform-based image codecs solve that by using a canonical base set of components that were not found by PCA. For example, basically all JPEG- and MPEG-developed image compressors use blocks of $2^k\times 2^k$ pixels, project them onto the base vectors of the 2D-DCT (or a defined set of 2D wavelets), and only encode the coefficients. You don't have to send the base functions (components) – the DCT is known to the receiver.
So, for a single image, PCA makes your data bigger, not smaller, and hence is useless.
For movies, where the same deconstruction of still images might be used multiple times in consecutive images, finding a base, transporting it, and then using it for many pictures, could make sense. But even in that case, on image-type data, PCA with a $m\ll N$ (which is what you would need to reduce data significantly) leads to very noticable artifacts; for example, consider these 32 frames (source: "Principal Component Analysis (PCA) applied to images", by Václav Hlaváč from Czech Technical University in Prague)
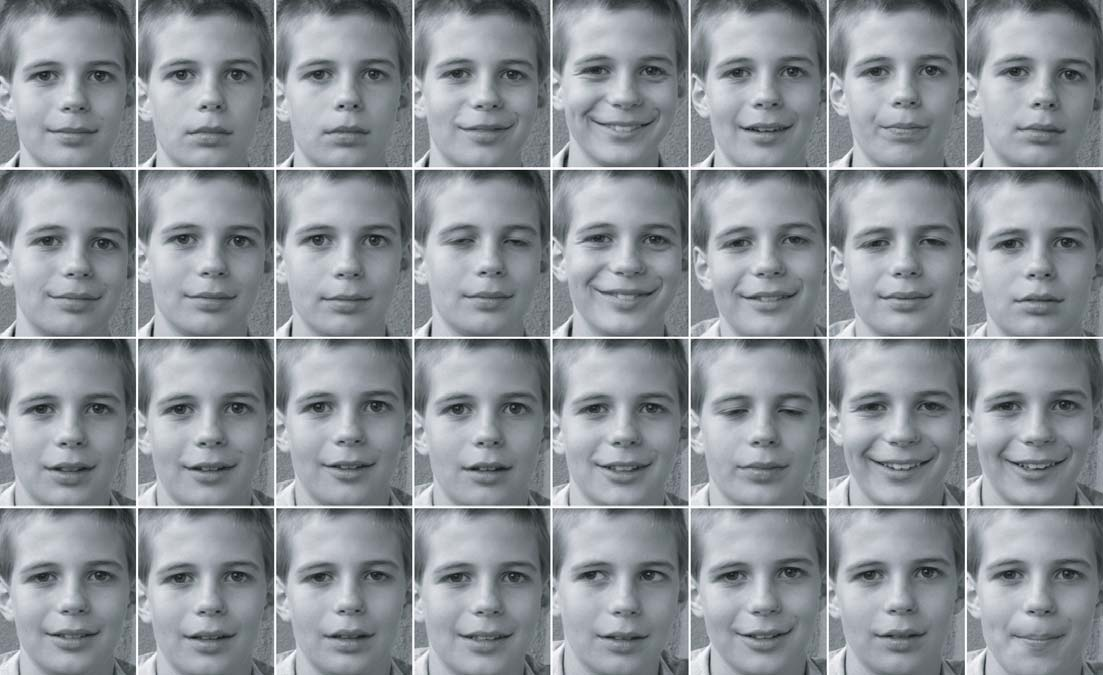
When we find the $m=4$ highest-energy principal components of that (still only a coefficient bits reduction by a factor of 8; even the 31 years old JPEG beats that trivially), and use them to reconstruct the 32 images, you get this:
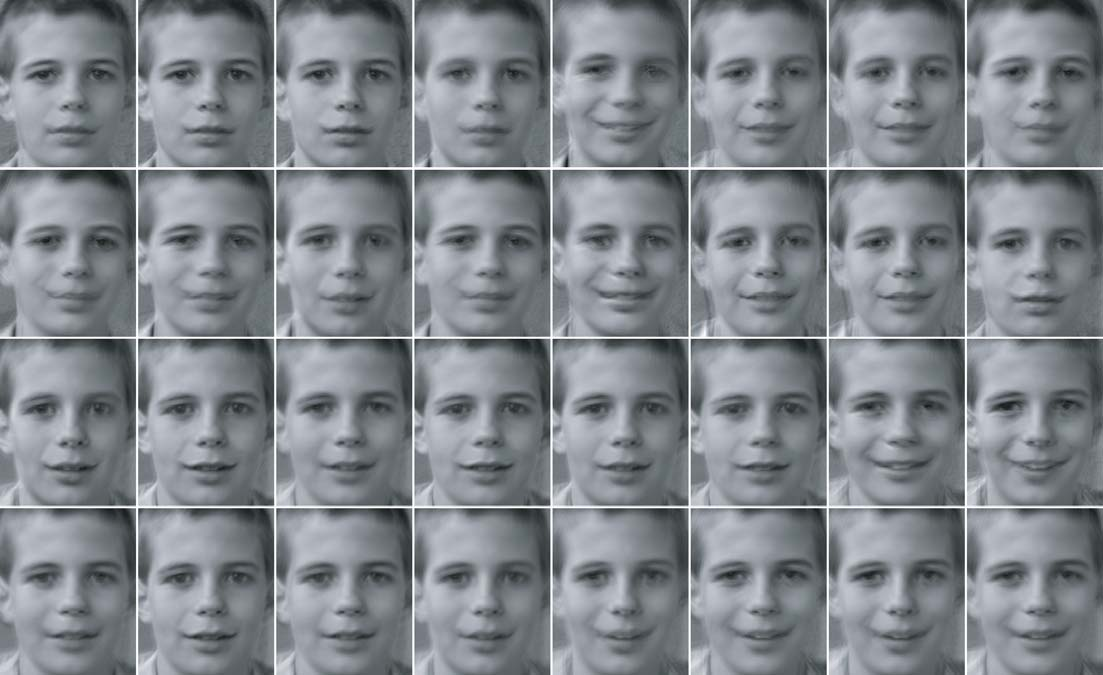
Observe the eyes: all eyes are "blurry half open". But the boy closed them, and reopened them. Look at the eyes in the middle of the bottom row: The boy looked to the side. PCA "removed" that information that is very important to the human looking at the pictures!
PCA directly on image data is really bad at preserving these kind of things, because it's in the end a linear operation on individual pixels, so you always get a pixel-wise average. Hence, even in the context of images that are very similar and where you hence would expect principal components to be shared, PCA is a bad idea. Video codecs use methods that are useful for human perception instead – for example, motion prediction. (There might be techniques to detect motion or similar frames through SVD; but these would work on the pre-transformed image, not on pixels.)
So, PCA solves none of the problems in image compression, but introduces more pixels to transport. It's simply in itself not useful.